Once upon a time, customers would walk into a store they knew to look for a product they needed. They would have a few stores top of mind, each mentally categorised according to the type of purchase they wanted to make. They would find an item on display which more or less matched their needs and called it by its official, store-created name. Similarly, online they might just type the name of their store into the address bar of their browser.
Those days are gone.
In today’s Google era, customers no longer stay faithful to one of the stores they know. They first think of what they want, describe it in their own words in their Google search, then try out more and more refined searches until then buy it from one of the first results in the Search Engine results page.
This has led to a dramatic shift for retailers. They can no longer expect their customers to look for their items based on what they call them. Instead, user language is increasingly the way to go.
The problem?
In order to ‘speak’ user language and be found online, stores need to align their content in every nook and cranny of their store. With thousands of products and hundreds of different ways of talking about any product, this turns into a mountain of time-consuming work. This led us to wonder: could we use AI and a rich external source of data to make the lives of retailers radically simpler?
Why is it so hard for retailers to give their products the best categories?
There are a large number of intuitive and natural ways to classify different products. The more complex a product is, the more ways there are to describe it — and these are all equally valid.
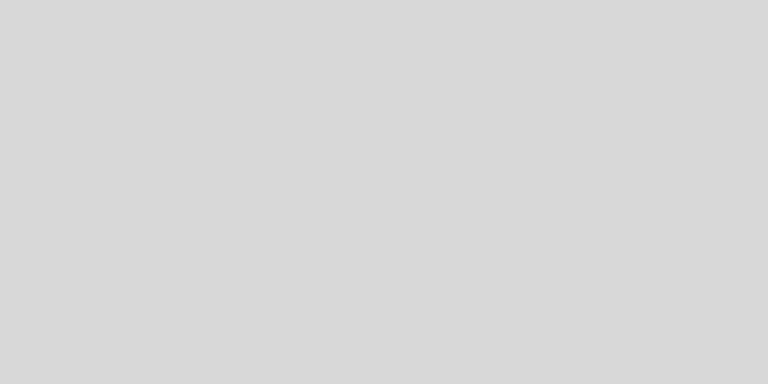
Take a pair of strappy sandals:
What would your friend call them? Mid heel sandals? Heels with straps? Summer heels? Or perhaps ankle-strap stiletto’s?
Different words or categories come more naturally to different audiences of customers. How users search shifts and changes. These changes are very swift and may depend on seasonality or occasion, on hype and trends.
For instance, ‘beach cardigan’ and ‘sweater blazer’ are very different categories which could designate the same item sold in different seasons or styled in a different way. Trendy categories such as ‘slides’ may get more traffic than, say, ‘sandals for men’. And if a huge player like Nike creates a Dri-FIT line of moisture-soaking apparel, you best believe this is a term for which users will be searching (even within other brands).
Although users’ vocabulary is wide and complex, retailer categorisation of a product is fixed. Retailers only have their own product data to play with, and it is, more often than not, insufficient. It can force them to think about their products in their own organisational way. Not only are they missing important categories which would make sense to users. This can be incredibly time-consuming for marketers who’d like a simple way of connecting their products to new audiences.
There’s an old car industry joke that you can see the organization chart of a car company in the dashboard, and also see that the steering wheel team hates the gear stick team.
Sometimes working at a retailer can be like that. Organisational thinking about what categories customers need hasn’t changed since the company was founded. The jacket & coats department rarely ever needs to talk to the sweaters & cardigans department. However, when customers want coatigans, it can be hard to respond with the right marketing angle.
Similar.ai’s Universal Product Ontology: the everyday knowledge of how customers think about products
We created the Universal Product Ontology. These includes thousands of tags or labels: all the ways in which customers think about products. We group those into different types, like shapes, colours, parts, styles, occasions, brands, materials and so on.
We also organised each of our thousands of labels into a set of overlapping taxonomies, as each label is interrelated to others in a particular way. For instance:
- A bandeau bra is a kind of bra, in turn a kind of lingerie, which is, in turn, a kind of clothing, which is a kind of fashion product. These are part of what we call our ‘shape’ taxonomy.
- Similarly, burgundy is a kind of red, which in turn is a type of colour, another taxonomy.
- The label group ‘collar’ can be associated with dresses, t-shirts, shirts and so on, and we have many different kinds of collars.
Our classification system makes sense of all these complexities. We call the Universal Product Ontology universal because it works for anything product-related. Universal Product Ontology is a bit of a mouthful, so we often refer to it as the ontology. It’s like a table of elements for product characteristics.
Any single product might have only have a dozen labels in the ontology or it might have thirty or more. More complex products with lots of different parts have more labels. A dress or a pair or shoes will have more labels than a pair of glasses or a spoon.
Fashion, homeware, beauty and holidays are some of the everyday domains which make people people. The attributes in these domains are not purely functional, but emotional and social too, and so it makes sense that the retail vocabulary we’ve built references all these experiences too. Our ontology is a knowledge graph which helps AI understand how people intuitively think about these verticals.
How we understand product pages and catalogues
Our product understanding technology looks at any product page and understands how a user would describe that product. It leverages both image recognition technology and natural language understanding to handle the product images and unstructured text you find on product pages. It turns a product page in to the labels in our ontology which succinctly describe how people would think about this product.
It does this whether or not these labels are characteristics the retailer’s own site understands or whether or not these are words in the product description. It does at the scale needed to process millions of product items in an hour, completely automatically.
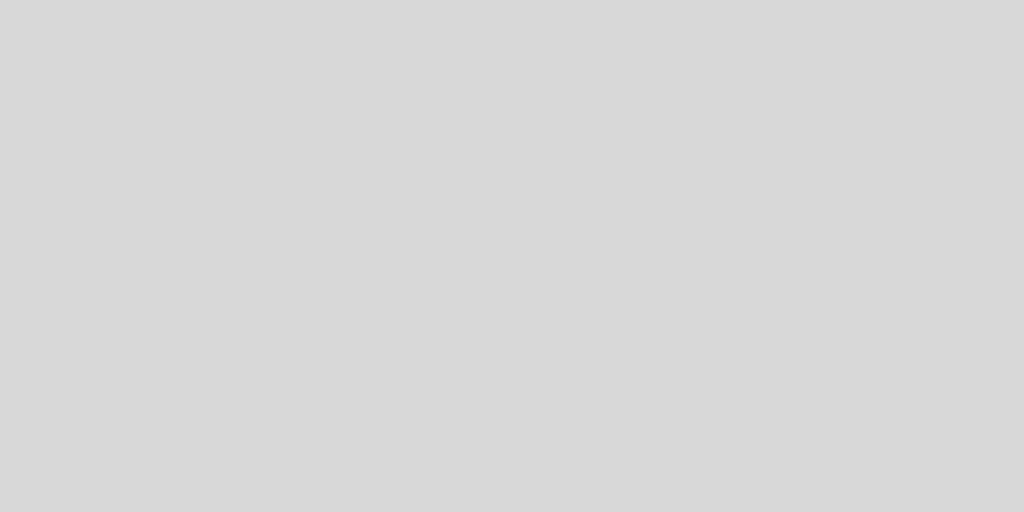
In this way, we can read in a product catalogue of millions of product items and turn it into the essential elements of how people think about these items. We can find the most important clusters in the catalogue.
How understanding how customers search helps Similar.ai get fluent in how to market products
If we could translate a product catalogue into all the ways in which a user might search for those products, we realised we could help retailers work out the best ways to market their products to new audiences. But data from a single retail store is not enough to understand how customers talk about products, even if we understand how customers think about products. We needed a source of a lot of users looking for products, so we turned to the categories users type in to search engines. We decided to partner with the search engine marketing analytics company SEMrush, which has petabytes of search engine marketing data, covering what people in different markets search for, which sites Google ranks as being relevant for those searches and how that changes over time. Taking on all this data is like drinking from the proverbial fire hose.
The challenge of matching retailer products with user language is a natural fit for Artificial Intelligence. But it is a challenge which needs tackling on each end, both product understanding and category understanding, in which we are able to derive the intent of the user doing the search. We used category understanding to build a special kind of search engine that matches products through the ontology to the categories customers might use to search for these products. The ontology acts as a lingua franca, or Rosetta Stone, to match between a product catalogue and the words users would employ to find those products.
What exactly is search intent?
Humans are quite good at understanding intent based on context and phrasing. Computers… less so. But what do we mean by intent in the context of search?
Imagine you are looking to purchase a Hugo Boss product and simply type ‘boss’ into Google. There are a few different results you may be offered:
- Hugo Boss products
- Local businesses with ‘boss’ in the name
- Boss guitar products
- Music videos with ‘boss’ in the title
- The Boss Study Association
- How to heal damage caused by bosses in video games
We use search intent to find the millions of times people search for ‘boss’ and are looking to buy clothing (or bags, shoes, accessories, homeware etc). Our top eCommerce categories for ‘boss’ in the domain of clothing look like:
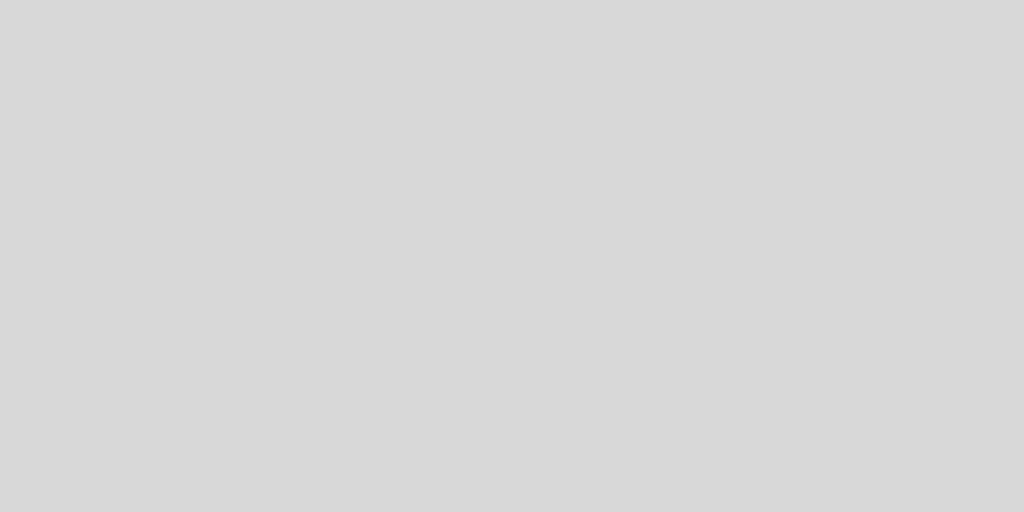
This filters out all the noise and lets retailers focus on what’s important: the ways in which people are looking for products in their domain.
Why is understanding user intent so useful?
To put it simply, we take a search query, zoom in on the relevant intent and translate that into our ontology. For instance, our platform understands when looking at ‘hugo boss jacket’, that the user is looking to buy a jacket from the brand Hugo Boss. Similarly, it sees when looking at ‘flowery mini dress’ that the user wants a dress, with a short length and a floral print pattern. Or, it can understand that when a customer lands on a category page about ’round wooden table’ that the user wants to buy a table with a round top made of wood. We can zoom in or out of detail.
There are lots of ways to use this. The most basic is that we understand which of the trillions of searches typed or spoken into Google, Amazon or Alexa each year are people looking for products.
We can also see how many people are interested in different item categories: fashion, homeware or beauty, say. Within fashion and apparel, we can see which of the searches correspond to people looking for or looking to buy clothes, shoes, bags or accessories. Each of these domains has its own universe of categories.
Search intent is almost the holy grail for marketers: it gets to who is looking to buy products. By understanding the intent of the searcher, we understand demand for any product category. At a more granular level, we can now see all the categories related to a specific retailer’s inventory in a certain market on a certain day — and can easily select the best categories with which they can market their products. At this level, seeing across thousands or millions of products, with million of categories, it’s common to uncover surprising opportunities to stand out in a market to unlock product revenues. Retailers have told us that seeing our category recommendation overview gave them fresh insights, because suddenly they were not seeing their products as defined by their own data, but seeing how their customers saw their product inventory. Retailers also value being able to understand demand intelligence pulled from this industry-wide level let them peek a little farther into their future demand for their different markets than their competitors.
Mining intent from search engine data related to a product inventory can help a retailer make data-informed decisions on how to best market their products to match upcoming demand.
Why is understanding search intent so hard?
Category understanding is a lot trickier than it sounds. Software struggles a lot with ambiguity. Imagine a user types the following search into Google: ‘red valentino bag’. This could mean a red bag from the brand Valentino, or perhaps any colour bag from the brand Red Valentino. Now what if the user searches for: ‘baby pink overalls’? Are they looking for overalls in the colour baby pink, or with pink overalls for babies?
Another obstacle for search intent interpretation is… people. More specifically, their tendency to bend the rules — especially when it comes to language. They use language they would never use in a formal situation, and which retailers would never think of using in their product descriptions. Even if they did think of it, in most cases they wouldn’t use that language because it simply doesn’t seem ‘proper’.
Of course, it’s not all about customers who search the same way as they talk. Product pages contain increasingly obscure language, perhaps because it gives a more luxurious feel. Which makes users feel like they aren’t buying just some simple product, but really the bee’s knees. Fancy restaurants are known for this; you can read about Black Aberdeen Angus Sirloin but can’t find any steak or see some Deep Battered Flaky White Atlantic Cod, served with a Small Platter of Garlic Infused Pan-Fried Melt-in-the-Moth Russet Jo-Jo Wedges when ‘fish & chips’ would do. This works when the customers are in the store and it was great when customers got to the store by knowing the name of the store. But it’s terrible when the customers find a store by typing the categories of products they are looking for.
Similar.ai has solved both of these problems — category understanding on the one hand and product understanding on the other — using our ontology of products to match between the two. We learned to love ambiguous data: we used AI to learn from the data itself. If customers intuitively understand the categories they use to search on Google, so does Similar.ai. If customers understand a product page, so does our platform.
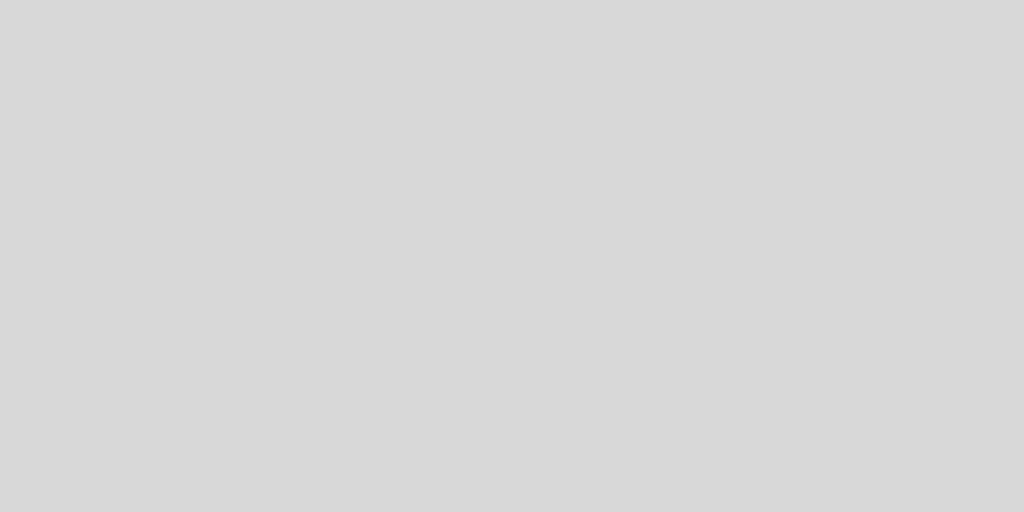
The future of product discovery is customer-centric
Retailers have more insight than ever into what customers want, and how they go about looking for it. Industry lingo takes up less and less space in consumers’ minds as they opt for the convenience of searching for what to buy using their own words. Product search and discovery is a conversation and in order to win at marketing their products, in order to speak to what customers are looking for, retailers need to be fluent in how customers express themselves and in how to differentiate themselves in those channels.
Article created by Robin Allenson, Co-Founder & CEO and Marianne Lalande, Marketing Manager at Similar.ai. Similar.ai offers retailers and marketplaces a platform to find the categories to unlock product revenue and quickly publish category pages.