Taking place yesterday at the Meltwater office in London, the DataScrum event brought back together the city’s alternative data community.
The event focused on the ways companies and data scientists can use alternative data to solve problems across industries.
Specifically, speakers dealt with the ways firms can leverage alternative data in financial models, but also how they educate the audience about their products and improve investment performance based on alternative data.
James Ritchie, Meltwater
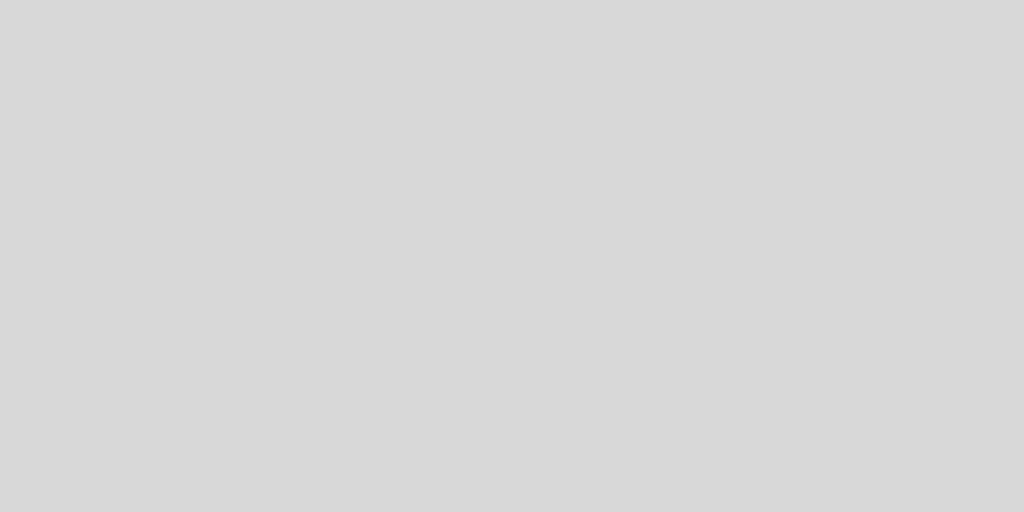
The event kicked off with James Ritchie, Senior Director at Meltwater, telling the community about the company’s latest updates.
What Meltwater is doing, Ritchie said, is trying to make data easier to consume.
To this end, the company is providing diversified and illustrative packaged data sets. They range from US Business News Sentiment to daily snapshots of all the potentially market-moving business events detected on its Signals platform and many others.
Ritchie explained that, while most of these data sets are tailored around finance, others may also be useful for applications in other sectors. For example, he mentioned a data set relating to sentiment and volume coverage for the 15+ of the top-selling drugs in the US Pharma market and one around each Premier League team and players.
The Meltwater executive concluded his presentation reminding the audience that the companies’ datasets are now on the newly opened AWS Data Exchange website.
Steve Muzzlewhite and Sayad Baronyan, EPFR
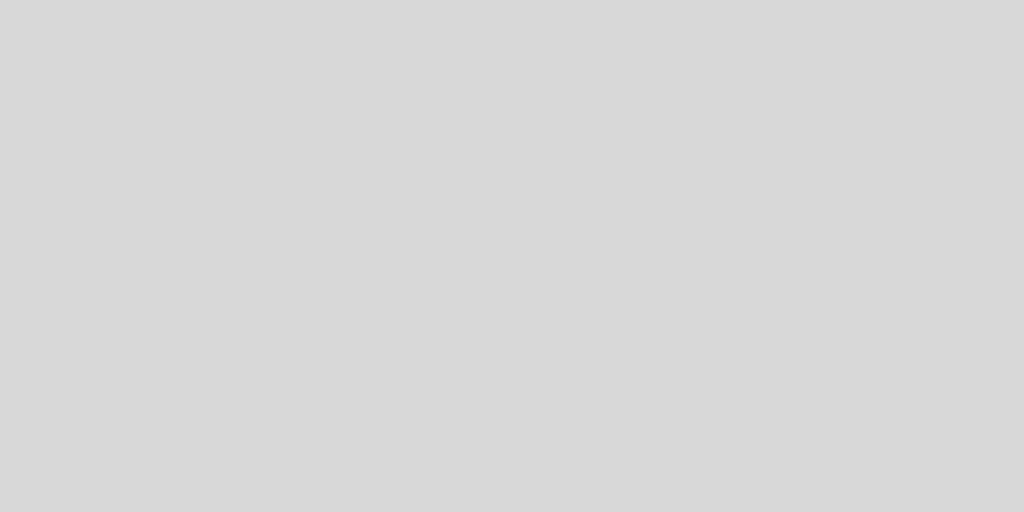
The event then proceeded with Steve Muzzlewhite and Sayad Baronyan from EPFR speaking about how financial institutions can use their services to manage fund flows and asset allocation data.
EPFR is part of Informa Financial Intelligence and ranks both traditional and alternative funds domiciled globally with $36 trillion in total assets.
Sayad, who is EPFR’s quantitative analyst, then showed the audience some of their systematic trading strategies. He stretched consistently that their company aims to show clients the data in an honest way.
The quantitative analyst finally explained how their platform ranks fund flows, and how it consequently is a very powerful tool to understand sentiment and act upon it.
Daniel Herde, CausaLens
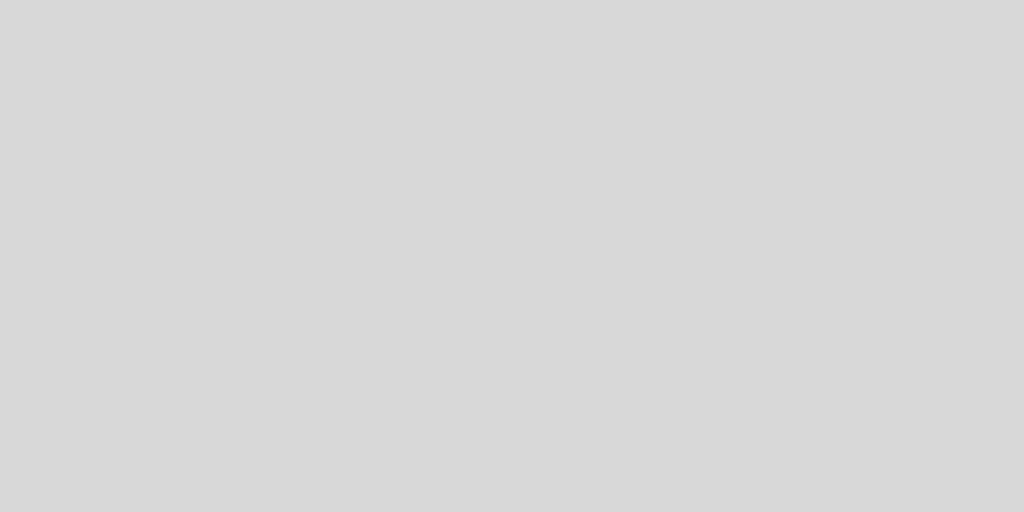
Data scientist Daniel Herde from CausaLens then took the mic.
According to its website, CauseLens has built “a machine that predicts the global economy in real-time.”
Herde conformed pretty much with this definition, highlighting the “fun of data manipulation” and saying that if companies generate enough data sets, they will eventually find the answer to their questions.
However, Herde also highlighted that different companies have different business lines. So he asked the audience: “how do you predict data accurately?”
The data scientist believes that, despite data providers trying to make life easier for their clients, they would be losing valuable signals.
He gave an example of how sentiment can impact stocks, and how missing crucial bits of information could render the data unusable.
The solution, Herde claimed, is to go from raw data to predictive signals in the most correct way as possible.
To this end, he said, CausaLens uses a fully transparent machine learning process, aimed at selecting and analyzing the most valuable raw data and turn it into valuable business-driven insights.
Linda Gruendken, GAM Systematic Cantab
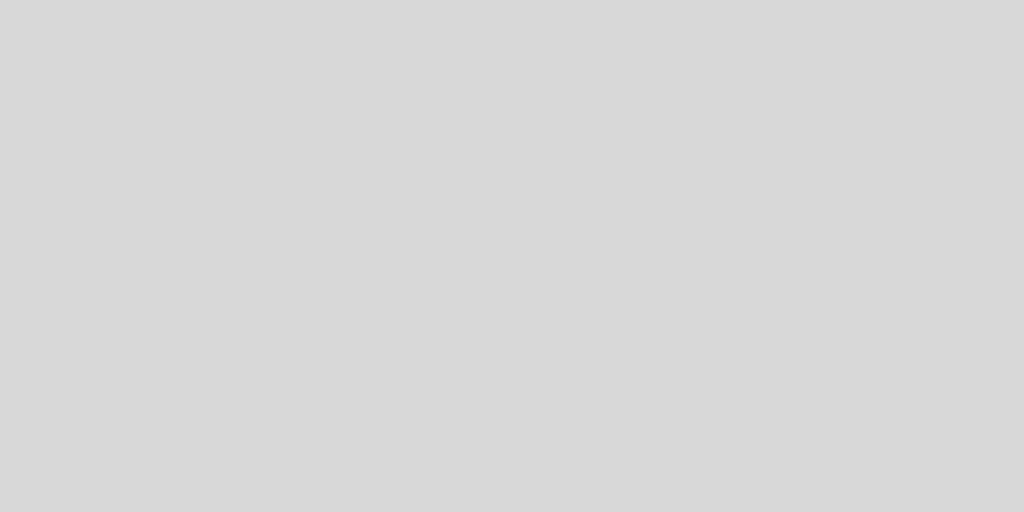
The DataScrum event then proceeded with the presentation by Dr. Linda Gruendken, a Senior Scientist from GAM Systematic Cantab.
Despite her technical background, Gruendken started by saying that, even as a data user, you can’t really not look at big data, as it permeates every aspect of our life.
She then proceeded to debunk the myths of machine learning, demystifying buzzwords like AI and machine learning, and claiming that a “magical robot” able to make good business decisions is just not going to happen any soon.
In other words, she explained, the quantitative investment process hasn’t changed drastically due to technological advancements but it has been enhanced by machine learning, and that’s why companies should use this technology optimally.
Gruendken suggested data scientists should focus on datasets that are useful to them now. Forward-looking data that is saying how risk is going to evolve.
She then showed the audience the way GAM finds alphas signals and how it classifies the resulting data based on different factors. How it tries to establish the size of their effect across different data sets.
The GAM scientist finished her presentation by bringing the public’s attention on two clear criteria for selecting data among the ocean of endless possibilities:
- How long to establish if a dataset is useful?
- How long to make that dataset useful after establishing it might be useful?
If both of these questions present positive answers, then a machine learning strategy can be formed which will act mostly autonomously, and that’s what GAM is all about.
Diana Golovko, SimilarWeb
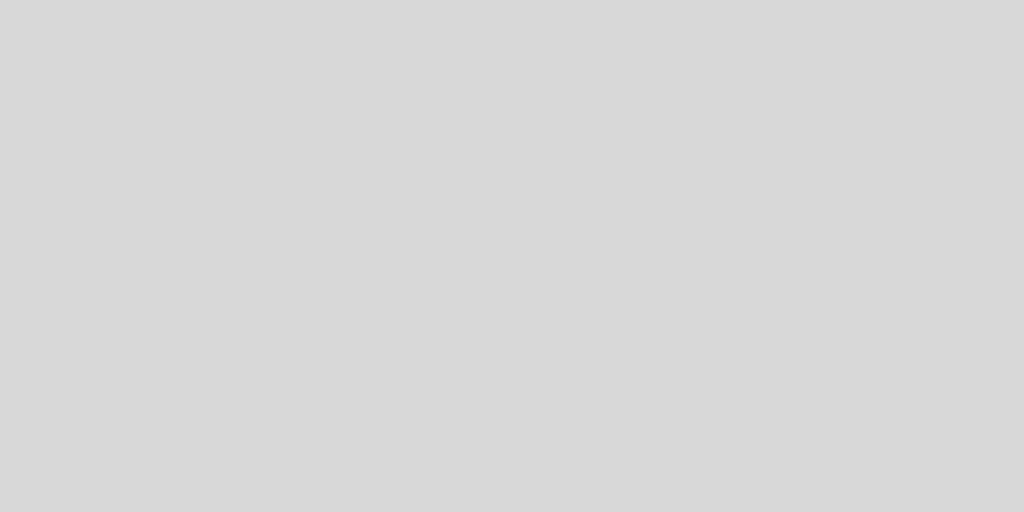
The last speaker at the DataScrum event was Diana Golovko, Senior Global Account Manager at SimilarWeb.
Golovko conducted a very much example-centered presentation.
She started by giving some figures about SimilarWeb and quoting the company’s mission: “to empower you with the insights you need to win your market”
After that, she quickly dived into two real-life examples where SimilarWeb’s data was used to connect apparently unrelated events and figures. The companies she spoke about were Netflix and Spotify, but the specific information belongs to the company, and cannot be posted on this blog.
Golovko then told the audience about the recent launch of four new vertical products and claimed that SimilarWeb has the largest digital panel globally.
She explained how they used data from Google and other platforms to calibrate their platform and once again stretched the importance of understanding datasets in context.
The evening then ended with some friendly networking and pizza. Were you there? Are you looking forward to the next alternative-data event? Follow our blog and DataScrum for updates.