Key Takeaway
Spotify is the largest on-demand music service application today. The firm has a record of pushing boundaries in technology by using AI and machine learning to enhance the user experience through nuanced customer data insights. They’ve recently acquired several data science companies to further push the envelope, ensuring they remain at the forefront of the music streaming world.
Why data is the magic ingredient for music streaming success
With tens of millions of users listening to music every minute of the day, brands like Spotify accumulate a mountain of implicit customer data comprised of song preferences, keyword preferences, playlist data, geographic location of listeners, most used devices and more.
Data drives decisions across every department at Spotify. This information is used to train algorithms which extrapolate relevant insights both from content on the platform and from online conversations about music and artists, as well as from customer data, and use this to enhance the user experience.
One example is ‘Discover Weekly’, which reached 40 million people in the first year it was introduced. Each Monday individual users are presented with a customised list of thirty songs. The recommended playlist comprises tracks that user might have not heard before, but the recommendations are generated based on the user’s search history pattern and potential music preference. Machine learning enables the recommendations to improve over time. Not only does it keep users returning, it also enables greater exposure for artists who users may not search for organically.
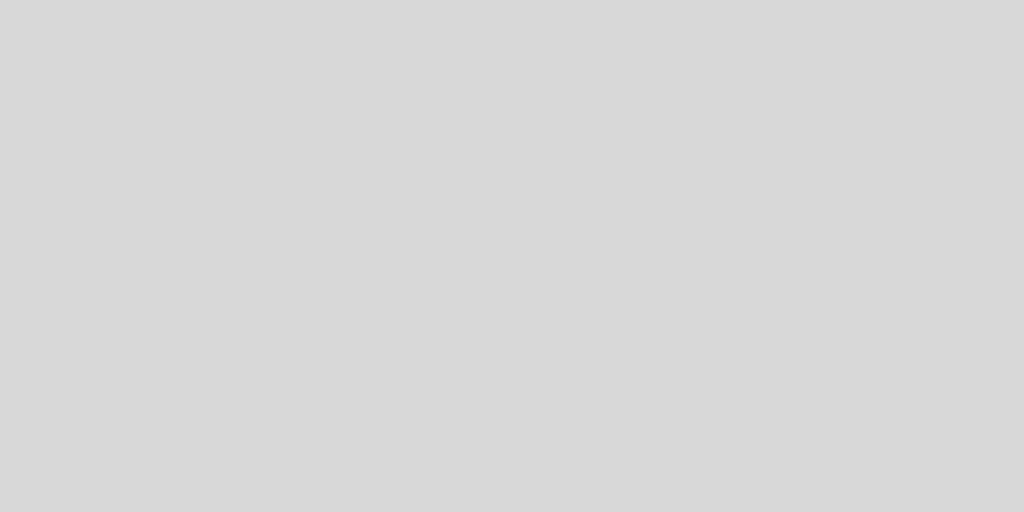
In order for Spotify to generate the ‘Discover Weekly’ personalized music list, the team uses a combination of three models:
Collaborative Filtering:
This involves comparing a user’s behavioral trends with those of other users. Content streaming platform Netflix similarly adopts collaborative filtering to power their recommendation models, using viewers’ star-based movie ratings to create recommendations for other similar users. While Spotify doesn’t incorporate a rating system for songs, they do use implicit feedback – like the number of times a user has played a particular song, saved a song to their lists, or clicked on the artist’s page upon listening to the song – to provide relevant recommendations for other users that have been deemed similar.
Natural Language Processing (NLP):
NLP analyses human speech via text. Spotify’s AI scans a track’s metadata, as well as blog posts and discussions about specific musicians, and news articles about songs or artists on the internet. It looks at what people are saying about certain artists or songs and the language being used, and also which other artists and songs are being discussed alongside, if at all, and identifies descriptive terms, noun phrases and other texts associated with those songs or artists.
These keywords are then categorised into “cultural vectors” and “top terms”. Every artist and song is associated with thousands of top terms that are subject to change on a daily basis. Each term is assigned a weight, reflecting its relative importance in terms of how many times an individual would attribute that term to a song or musician they like. Spotify doesn’t have a fixed dictionary for this, but the system is able to identify new music terms as and when they come up – not just in English, but also in Latin-derived languages across cultures. Of course, spam and non-music related content is discarded through a filtering process.
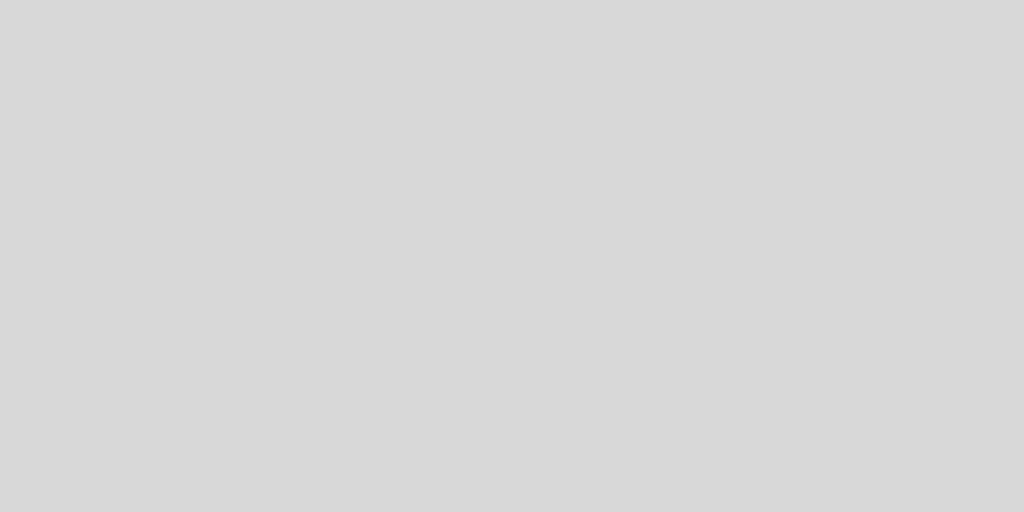
Brian Whitman, data scientist and founder of Spotify-acquired music intelligence company The Echo Nest, explores these models in further detail here.
Audio models:
Audio models are used to analyse data from raw audio tracks and categorize songs accordingly. This helps the platform evaluate all songs to create recommendations, regardless of coverage online. For instance, if there is a new song released by a new artist on the platform, NLP models might not pick up on it if coverage online and in social media is low. By leveraging song data from audio models, however, the collaborative filtering model will be able to analyze the track and recommend it to similar users alongside other more popular songs.
Spotify has also adopted convolutional neural networks, which happen to be the same technology used for facial recognition. In the case of Spotify these models are used on audio data instead of on pixels. Sander Dielman, a data scientist at Google, explains the technology further in his blog post.
In this way, Spotify portrays itself not just as a platform for popular existing musicians, but also one that provides opportunities for the next generation of budding musicians to gain recognition.
So how does Spotify know you so well?
Personalisation is a key element that contributes to Spotify’s superior user experience, and this is evident in the introduction of playlists like ‘Discover Weekly’ and ‘Release Radar’. But how does it know a user’s preferences so well?
In 2017 alone Spotify went on an acquisition spree to improve the technology behind their personalisation elements. One significant acquisition was French startup firmNiland which is self-described as “a music technology company that provides music search and discovery engines based on deep learning and machine listening algorithms.”
This was instrumental for Spotify as it led to service improvements for music listeners, leveraging Niland’s API and machine learning algorithms to generate better searches and music recommendations, and enabling users to discover the music they like more easily.
Spotify has also acquired blockchain company Mediachain Labs. This acquisition helps the right people get paid for every track played on Spotify – a task that would only increase in complication as the user base expands exponentially.
Blockchain technology is one of the most popular topics in the music business, as it’s one of the more innovative ways of making sure that transactions are processed more efficiently. The music industry’s transition from the sale of CD’s to MP3 downloads, and now streaming, has made it difficult to keep track of the trillions of data points that are required to make the correct royalty payments. Mediachain, in this case, is seen as a potential savior for the industry, not only to make the process more transparent, but also to make it more efficient.
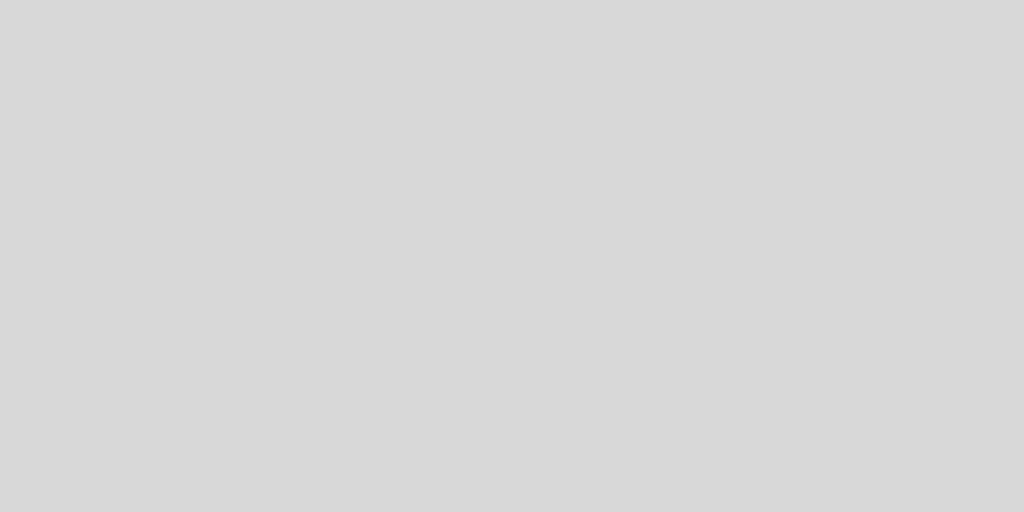
Machine learning, fueled both by user data and by external data, has become core to Spotify’s offering, helping artists to better understand their audience and reach and to get discovered, while helping Spotify remain on top of the music streaming space through a deep understanding of their customer base and predictive recommendations that keep users coming back.