Key Takeaway
Global fashion search platform Lyst is looking to transform smart search by using AI and machine learning to better understand how humans realistically search for fashion products. Leveraging its proprietary search data, along with external data from search and social media, the team also generates predictive insights in the form of brand and product hot lists, upcoming trends and more, informing business decisions for stakeholders across the industry.
Lyst enables fashion seekers to search thousands of online stores in one place, bringing together 5M products from 12,000 of the world’s leading brands. Last year over 70M shoppers from 120 countries started their search on Lyst.com.
The firm recently raised a $60M funding round led by LVMH, following which LVMH chief digital officer Ian Rogers will be joining the Lyst board.
The key to the platform’s success lies in its advanced search capabilities, which enable shoppers to search on the site in a way that actually makes sense for the user. Deep AI and machine learning advancements enable users to find products by entering search terms like “Kardashian dress” or “job interview” – rather than relying on pre-tagged keywords often used in the e-commerce space.
We spoke with Lyst Communications Director Katy Lubin about the data that drives Lyst, how the brand is combining internal and external data insights to form fashion predictions and index the world’s hottest products, and what’s next for smart search.
How it works: data-driven from day one
“Data and understanding of data is fundamental to Lyst,” Lubin told us. “We don’t hold any inventory or sell anything ourselves; as a platform we’re all about organising the huge amounts of retailer data from our partners in a way that our customers find easy to understand and navigate. That means describing our products in a consistent way and choosing which products appear where and when. We use data to get to the heart of what our customers want.”
As a result, the data scientists at Lyst are experimenting with advanced machine learning models to improve their search functionality, in an effort to create an experience that more closely mirrors the way humans realistically search for and talk about products.
“Training a supercomputer to be a fashionista is no mean feat; the fashion landscape is complicated and transient, and the trend lines and brand markers are constantly shifting and blurring.”
According to Lubin, most sites still use the ‘machine’ way of searching by which products are tagged with common industry terms and descriptors (i.e. t-shirt, blue, long sleeve). But in reality, that’s not how people search.
“Instead, I might type in ‘French girl stripey tee’ for example. We need to bring together how people search and how computers interpret that query. Fashion language is crazy. There are so many different words to describe things, and each of these terms can mean so many different things to different people. For a computer to understand that and understand that person’s intention when they search is a challenge.”
The Lyst Index: AI-Driven models predict fashion’s next big trends
As they continue to discover insights within the data they explore, the team has found a way to position themselves as leaders in trend forecasting, offering these insights through regular reports and indexes.
Each quarter, the Lyst team, in partnership with Business of Fashion, produces what they call the Lyst Index – a ranking of the hottest fashion brands and products globally. The index is produced via an AI model that has traditionally incorporated global Lyst search data, global Google search data, as well as engagement rates and conversion rates for products and brands worldwide over a 3 month period.
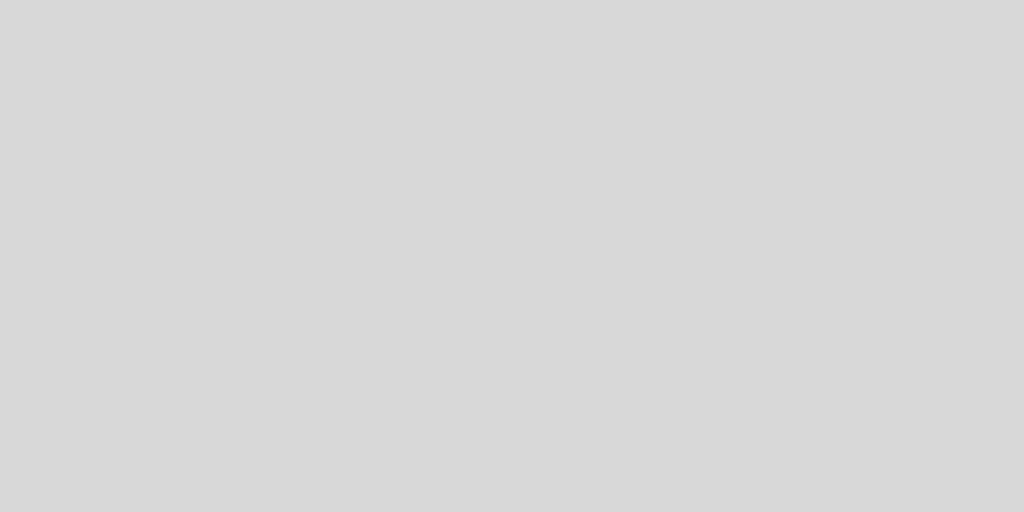
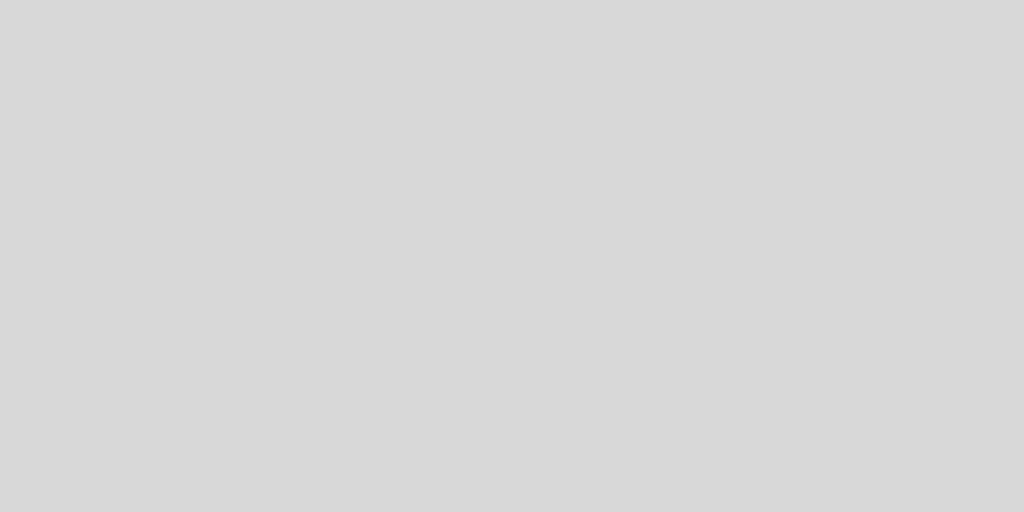
“Because it’s a quarterly report – there are relevant insights people can act on in a timely way. We’re so fortunate that we have access to this incredible data from 5M products worldwide,” Lubin said. The index is designed to be brand agnostic, so there is no risk of bias.
The next ranking, to be released in October, will also include external data from social media for the first time, integrating Instagram statistics into the model.
On the value of data from outside the brand’s firewalls, Lubin noted: “External data gives you a much more rounded view. Social media, for instance, offers a major signal of how hot something is. I’ve always been of the opinion that Lyst data is brilliant, but it doesn’t exist in a vacuum. Something like fashion is so reliant on external forces and drivers, so combined with data from Lyst it becomes so compelling compared with others.”
In addition to the quarterly index, the team compiles monthly trend predictions based on both their search data and social data. Each month’s data-driven street style predictions include both specific products from leading brands, like Prada’s neon clutch, and general trends like chunky trainers and anklets.
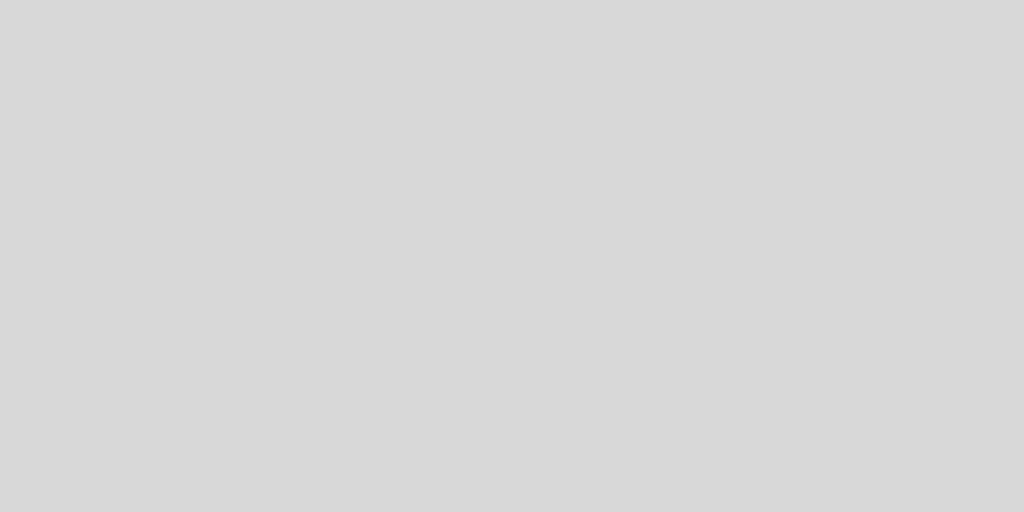
Deepening the connection between fashion search and popular culture
“We’re the intersection of where fashion and culture meet and impact one another.”
Ian Rogers initially met Lyst co-founder and chief executive Chris Morton while they were both working in the music industry. Rogers compares Lyst efforts in predictive search to what is happening in music, particularly on Spotify, where culture and lifestyle is becoming more intertwined with user behavior and consumer preference.
“If music is a leading indicator for consumer behaviour and distribution online, then I think that giving people help with finding what they want is really important,” Rogers said to the Business of Fashion. “There are tens of billions of songs on Spotify, and there are millions of SKUs on Lyst — and when you have that scale of availability, human beings need some help getting through that.”
It’s become common to see the news and events that are trending in media and social reflected on Spotify. Lubin explains, “When David Bowie died, for instance, you saw that people went and listened to his music. It’s the same with fashion. Anything from Kim Kardashian wearing a belt with the Fendi logo, to a major snowstorm in New York, can skew the data. We need to take fashion out of the bubble it exists in and put it in this larger social context of what the world wants to wear and why. It’s an interesting space to play with, and data can help us explore it.”
We need to take fashion out of the bubble it exists in and put it in this larger social context of what the world wants to wear and why.
Turning predictive insights into action
In addition to producing significant reports on trends and preferences, the Lyst team can observe data in a more reactive way to determine results. “For instance, what has a recent marketing campaign done for Nike, or Tom Ford, or are people interested in particular products?”
These forward-looking insights are useful for informing everything from merchandising decisions to geographical trends to watch. “This information can be used across any department – marketing, merchandising, strategy, investment. We do a bit of social targeting with our content to see who finds it interesting and relevant. The types of people that subscribe range from consultants and finance professionals, to ad agencies, to people working across all levels in the brands. It is relevant to most people in this industry, and to other stakeholders.”
The team continually finds new ways to feed insights back to their partners so that they can use this data to inform forward-looking decisions. “We use our data to better identify potential new customers and to create content that will be most relevant and resonant for our existing users. We also share our fashion data with the media to tell the stories of what the world wants to wear. It’s our most valuable PR asset, and publishers come to us for stats and reports. We love to dig into how popular culture affects fashion search and explore the reasons why.”
Search data is an incredibly powerful form of Outside Insight – it can demonstrate rising trends, uncover connections customers are making between products and culture, reveal where and when brands and items are trending and potentially indicate where gaps in the market may lie undiscovered. Combined with sentiment and engagement on social media, this data offers key consumer insights that can and should inform decision-making across all areas of an enterprise.