One of the core use cases for AI, given access to increasingly diverse datasets and ever-more data points, is to enable us to draw correlations and find patterns within data that were previously impossible to find with human analysis.
In the healthcare space there remain endless unknowns that can be solved with the help of AI-driven data analysis. Additionally, automation of expensive or time-consuming processes can not only save on costs but also valuable time in emergency situations, enabling us to hopefully save lives in the process.
According to Forbes, the total public and private sector investment in healthcare AI is expected to reach $6.6 billion by 2021. Technological developments resulting from this spend lead to significant cost reductions: Accenture predicts that top AI applications may result in annual savings of $150 billion by 2026. This has the potential to affect what’s known as the ‘iron triangle’ in healthcare – access, affordability, and effectiveness.
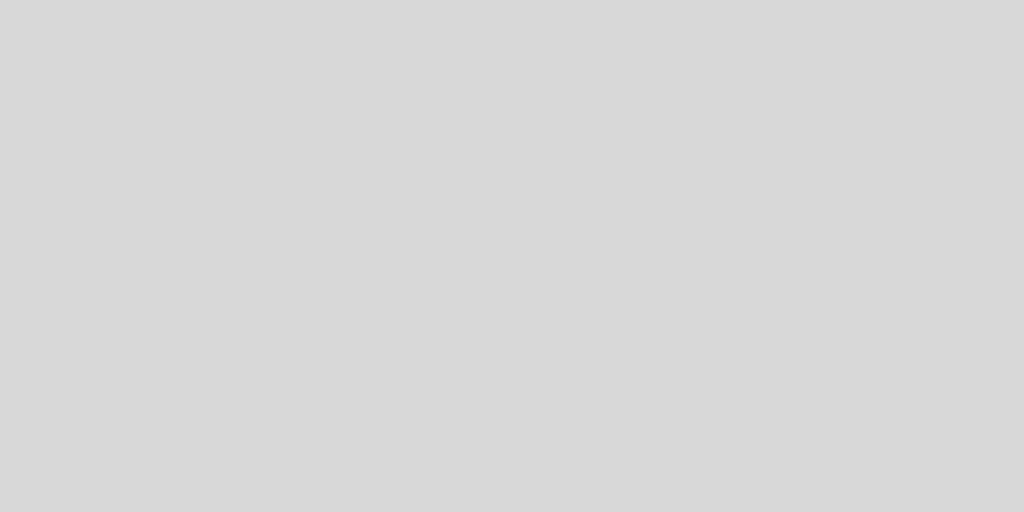
At Stanford University’s recent Human-Centered AI conference, Bill Gates spoke of AI’s potential particularly in the health and education space. “It’s a chance to supercharge the social sciences, with the biggest being education itself,” he said. Gates referenced ongoing studies in which data from customers using genetics testing company 23andme had been analyzed, leading to the discovery of an association between a shortage of the element selenium and incidence of premature births in Africa.
“Previous research has suggested that about 30 to 40 percent of the risk for preterm birth is linked to genetic factors. This new study is the first to provide robust information as to what some of those genetic factors actually are,” said Louis Muglia, MD, PhD, who coordinated the study and is the co-director of the Perinatal Institute at Cincinnati Children’s and principal investigator of the March of Dimes Prematurity Research Center.
Any previous studies that looked at this data were too small to significantly detect any real genetic variants. But thanks to AI and data from 23andme, “researchers were able to overcome those limits…using aggregated, de-identified data from 45,000 female 23andMe customers who consented to research.” These studies will enable further insights into human pregnancy and help to develop new preventative strategies for premature birth.
Tracking the spread of infectious disease
Our aggregate data points across external sources, from search history to use of consumer apps like smart thermometer Kinsa, also offer doctors and scientists the ability to understand how contagious illnesses specifically are spreading across a geography.
Where we previously relied on reports of flu-like illness from organizations like the Centers for Disease Control and Prevention (CDC), today with access to alternative data we’re able to predict the movement of these illnesses in real time, which enables public health agencies to engage in much more effective decision-making.
A new study released by Shaoyang Ning and Shihao Yang of the Department of Statistics at Harvard University proposes “a novel method named ARGO2 (2-step Augmented Regression with Google data) that efficiently combines publicly available Google search data at different resolutions (national and regional) with traditional influenza surveillance data from the Centers for Disease Control and Prevention (CDC) for accurate, real-time regional tracking of influenza.”
This method would enable those monitoring this data to also incorporate additional information from other sources and resolutions, “making it a powerful tool for regional influenza tracking, and potentially for tracking other social, economic, or public health events at the regional or local level.”
In 2017, a team from Northeastern University developed an algorithm that uses Twitter data for similar predictions, leveraging keywords related to flu. According to CNN, this “could help public health agencies plan ahead to distribute medical resources. They could also start campaigns earlier to encourage people to get a flu shot or take other preventative measures.”
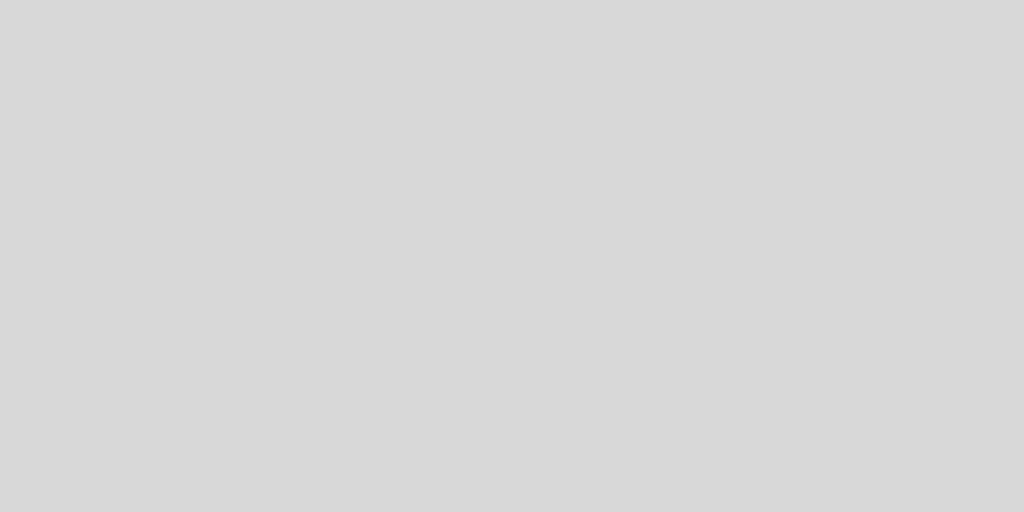
Other areas, from insurance prices, to treatment approvals, to billing and patient engagement are being impacted by the adoption of AI and external data, increasing transparency and eliminating human bias in critical decisions.
According to the Wall Street Journal, insurance company Anthem Blue Cross, for example, is looking to develop fair, interpretable AI that “in the future could justify rates and pricing to the public and to regulators, back up medical diagnoses used to approve or deny coverage, and provide evidence in claims denials or disputes.”
Even medication R&D is being impacted by AI, as companies try to mitigate the traditionally age-long approval process for new drugs by using AI to better identify which compounds are likely to succeed based on early-stage clinical data. “AI gives us a higher probability of obtaining success, even if we have some failures,” said Dan Rothman, chief information officer at Roivant Sciences. “It gives us more ‘at bats.’ There’s a lot of value to be found in making the drug development process more efficient.”
As we access, analyze and link more data points from external sources, using AI to find anomalies and help automate and digitize what are currently antiquated processes, the potential for AI to change the healthcare industry is immense – but many argue that so too is the responsibility to approach any applications with regulation, an eye toward potential bias and human judgment.